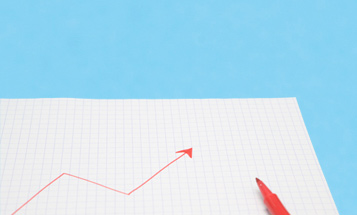
“The numbers don’t lie.”
You hear that all the time. Even if it’s mostly true, the numbers can be slippery, cryptic, and, at times, two-faced. Whether they represent findings about your customers, products, or employees, they can be maddeningly open to interpretation.
With big data getting ever bigger and infiltrating more and more parts of your business, the need for ways to understand the numbers’ meaning is becoming more acute. As Tom Davenport of Babson College and MIT writes in the current issue of HBR, companies must “fundamentally rethink how the analysis of data can create value for themselves and their customers.”
In this series, “From Data to Action”, researchers and practitioners explore the fast-changing landscape of data, show how you can learn to tune out most of the noise to focus on the all-important signal, and translate your new knowledge into bottom-line improvements. Scott A. Neslin of Dartmouth’s Tuck business school puts it perfectly when he says the numbers tell you a lot of stories simultaneously—the trick is figuring out which one really matters.
Neslin’s case in point concerns customers who haven’t bought for a while—one set of data tells you they’re not worth pursuing, while another says they are; which story is right? Susan Fournier of Boston University and Bob Rietveld of analytics firm Oxyme ask a related question: Is big data enough? Aggregate numbers can tell you a lot, but they say very little about how individual customers are thinking and talking about your products.
In a business-to-business context, Joël Le Bon of the University of Houston takes the question further, pointing out an often-overlooked, small-data source of useful information about your competitors’ plans and schemes: Your sales reps. He explains how to cultivate a strong flow of intelligence that can have a powerful impact on strategy.
In other articles, we’ll move beyond specific cases to get at the broader question of how managers can learn to pick the most important kernels of knowledge from the rush of data. Is it critically important for companies’ consumer-data teams to have someone who truly understands statistics in a leadership position, or can an untrained manager learn to be more discerning about data?
And are there times when you should completely ignore the data and go with your gut?
After all, if companies often fail to analyze data in ways that enhance their understanding and neglect to make changes in response to new insights, as Jeanne W. Ross and Anne Quaadgras of MIT and Cynthia M. Beath of the University of Texas say in the current HBR, then what’s the point of paying big money for big data?
While recognizing companies’ struggles to make sense of information, Ross et al argue that what really matters more than the type and quantity of the data is establishing a deep corporate culture of evidence-based decision making. That means establishing one undisputed source of performance data, giving all decision makers feedback in real time (or close to it), updating business rules in response to facts, and providing coaching for decision makers. It also means encouraging everyone in the organization to use data more effectively.
The sentiment about including everyone in the company in the use of data is echoed by McKinsey: When business-unit leaders invest in training for managers as well as end users, “pushing for the constant refinement of analytics tools, and tracking tool usage with new metrics,” companies do eventually transform themselves, bringing data-driven thinking into all aspects of the business and fulfilling data’s vast potential.